3D Vision – NeRFs & INRs

Course Description
In this course, “3D Vision – NeRFs and INRs”, you will learn about the basics of 3D Vision and how to use state-of-the-art 3D vision algorithms such as Neural Radiance Fields, Gaussian Splats, and Implicit Neural Representations.
In this course, you will engage in hands-on activities, homework, and instructor consulting to make learning 3D Vision enjoyable and rewarding. You will also be able to tackle real-world scenes for reconstruction and rendering. By the end of this course, you’ll have the skills and confidence to reconstruct any scenes from your smartphones and render them.
This course is one of 6 courses in the Advanced AI Techniques pilot Micro-Credential pathway offered by the Translational AI Center at Iowa State University.
Complete any 3 courses listed below to earn the Advanced AI Techniques badge:
- Scientific Machine Learning (SciML)
- Graph Neural Network
- Self-Supervised Learning
- Parallelism in Deep Learning
- 3D Vision – Nerfs & INRs
- Reinforcement Learning
Learn more about Micro-Credentials at Iowa State University!
Prerequisites
- Basic Python programming
- Basic understanding of deep learning and computer vision
- Basic PyTorch programming
Intended Audience
The course is intended for a broad audience within the spectrum of the software and technology industry, including software engineers, data scientists, data engineers, data analysts, research scientists, and software developers. The course is designed to provide a basic understanding of 3D Vision in Deep Learning and how to use these models for simulation, visualization, and, analysis.
Learning Outcomes
Assessments
Course Outline
By the end of the course, you should be able to:
- TBD
- 2 Quizzes to learn basic knowledge of 3D Vision and the different INR and NeRF algorithms.
- 1 exercise in which you will set up a computing environment for NeRFStudio based on hands-on activities.
- 1 Coding assignment to reconstruct a scene captured from a smartphone.
- Module 1: TBD
Course Procedures
The course starts on March 31, 2025. All coursework must be completed by May 31, 2025, in order to earn the micro-credential badge. You will continue to have access to the course materials until January 1, 2026. The approximate time to complete this course is 16 hours.
This course has an instructional period from March 31 to April 27, 2025. During this instructional period, course materials will be released weekly and live synchronous sessions will be held. You may complete the course materials at your own pace. Live Zoom meetings will be conducted for interactive coding sessions. A suitable time for these live sessions will be determined through a group poll. The recordings of those sessions will be available soon after each meeting.
You will receive the 3D Vision NeRFs & INRs micro-credential badge upon successful completion of the course assessments.
Course Materials
Course materials are provided within the course. No additional purchase is required.
Contact Information
Contact isopd@iastate.edu for more information.
Course Developer
The Translational AI Center breaks down disciplinary silos to bring together core Iowa State artificial intelligence researchers and subject matter experts interested in applying new technologies to their work. For more information, visit Translational AI Center at Iowa State University
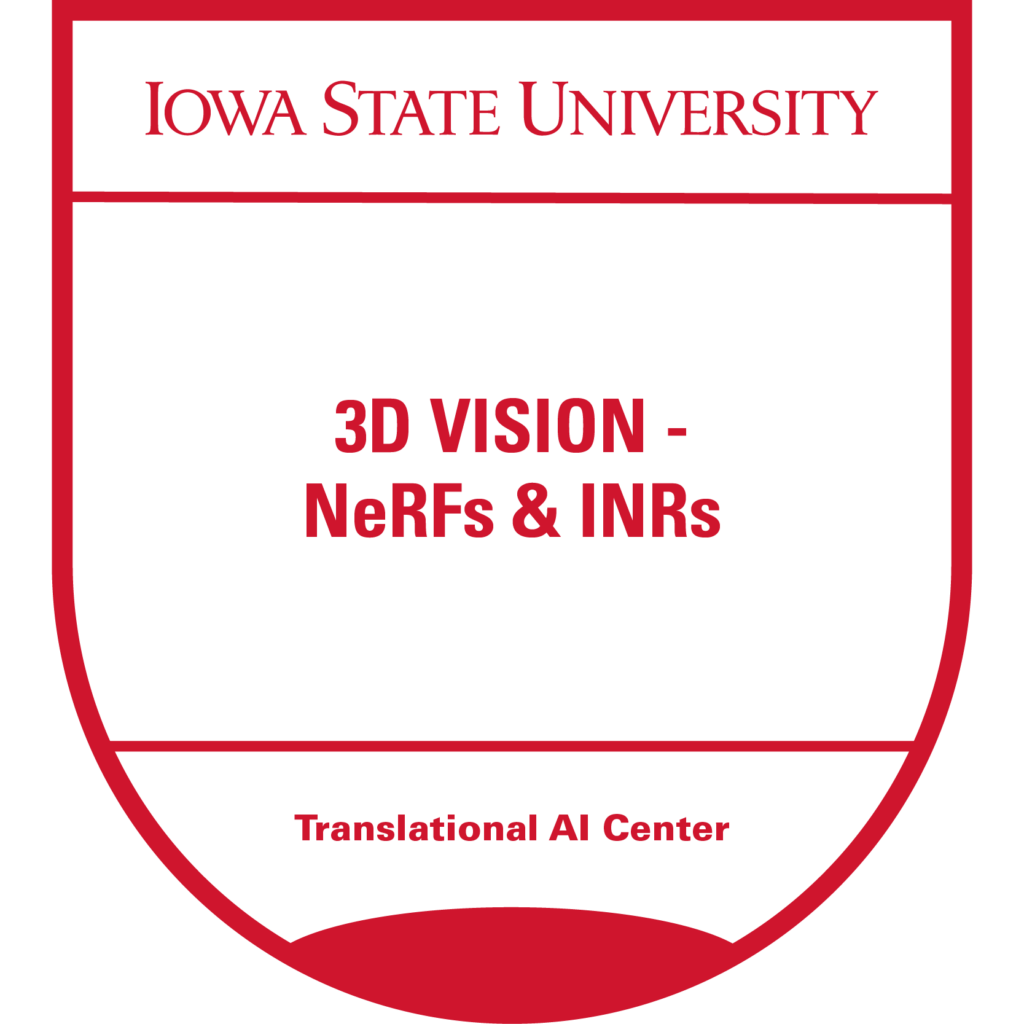
At a Glance
Registration Cost: $750.00 $500.00 USD (Initial Promo)
*$300.00 Student Discount Available
Course Hours: 16 hours
Course Start Date: March 31, 2025
Last Day to Register: March 24, 2025
Instructional Period & Live Sessions: March 31-April 27, 2025
Last Day to Earn a Micro-Credential Badge: May 31, 2025
Time to Complete Badge: 61 Days
*At the time of registration, you’ll be asked to create an account for this course. Use “.edu” email address for a student discount. $250.00 will be immediately credit back after purchase.
Instructor
Zaki Jubery, Research Scientist
Zaki Jubery is a research scientist in the Translational AI Center (TrAC) at Iowa State University. His research interests are in (i) High-throughput phenotyping (ii) Crop modeling (iii) Image processing (iv) Applied machine learning in agriculture.
Zaki works on integrating engineering tools into various agricultural applications. He has been dedicated to pioneering research in this field since September 2013.
Zaki earned his Ph.D. in Mechanical Engineering from Washington State University and completed a postdoctoral fellowship at the University of Illinois Urbana-Champaign. Before transitioning to agriculture, his background includes designing, simulating, and manufacturing point-of-care microfluidics sensors for biomedical and industrial applications.