SciML – Scientific Machine Learning

Registration Reopens in Fall 2025
Master SciML: Transform Theory into Real-World Solutions with PyTorch!
Course Description
Dive into the theory, implementation, and limitations of Scientific Machine Learning (SciML) models. In this course, you will engage in hands-on and practical activities under the expert guidance of our experienced instructors. Learning SciML will be enjoyable and rewarding as you engage in real-world applications, such as solving partial differential equations using physics-informed neural networks (PINNs) and neural operators (PyTorch implementation). By the end of this course, you’ll not only understand the theory behind SciML but also have the confidence and expertise to tackle any partial differential equation with PyTorch.
This course is one of 6 courses in the Advanced AI Techniques pilot Micro-Credential pathway offered by the Translational AI Center at Iowa State University.
Complete any 3 courses listed below to earn the Advanced AI Techniques badge:
- Scientific Machine Learning (SciML)
- Graph Neural Network
- Self-Supervised Learning
- Parallelism in Deep Learning
- 3D Vision – Nerfs & INRs
- Reinforcement Learning
Learn more about Micro-Credentials at Iowa State University!
Prerequisites
- Basic Python programming
- Basic understanding of numerical methods and deep learning
Intended Audience
The course is intended for a broad audience within the spectrum of the software and technology industry, including software engineers, data scientists, data engineers, data analysts, research scientists, and software developers. The course is designed to provide a basic understanding of AI and how to use PyTorch for a broad range of audiences.
Learning Outcomes
Assessments
Course Outline
By the end of the course, you should be able to:
- Apply scientific machine learning (SciML) models to solve partial differential equations (PDEs) for engineering problems.
- Implement Physics-Informed Neural Networks (PINNs) using PyTorch to solve differential equations.
- Analyze the theory, implementation, and limitations of neural operators.
- 2 quizzes to help design and debug SciML models (there will be unlimited attempts).
- 2 coding exercise questions, which include implementing Python codes based on hands-on activities. This includes coding a simple physics-informed neural network and using state-of-the-art neural operators (FNO, DeepONet) to learn partial differential equations (PDEs) from data.
- Module 1: Introduction to Partial Differential Equations (PDEs)
- Module 2: Physics-Informed Neural Networks (PINNs) Codes
- Module 3: Operator Learning (Fourier Neural Operator)
- Module 4: Operator Learning (DeepONet)
Course Procedures
The course starts on September 6, 2024. All coursework must be completed by October 31, 2024, in order to earn the micro-credential badge. You will continue to have access to the course materials until August 31, 2025. The approximate time to complete this course is 16 hours.
This course has an instructional period from September 6 to September 30, 2024. During this instructional period, course materials will be released weekly and live synchronous sessions will be held. You may complete the course materials at your own pace. Live Zoom meetings will be conducted for interactive coding sessions. A suitable time for these live sessions will be determined through a group poll. The recordings of those sessions will be available soon after each meeting.
You will receive the Scientific Machine Learning (SciML) micro-credential badge upon successful completion of the course assessments.
Course Materials
Course materials are provided within the course. No additional purchase is required.
Contact Information
Contact isopd@iastate.edu for more information.
Course Developer
The Translational AI Center breaks down disciplinary silos to bring together core Iowa State artificial intelligence researchers and subject matter experts interested in applying new technologies to their work. For more information, visit Translational AI Center at Iowa State University
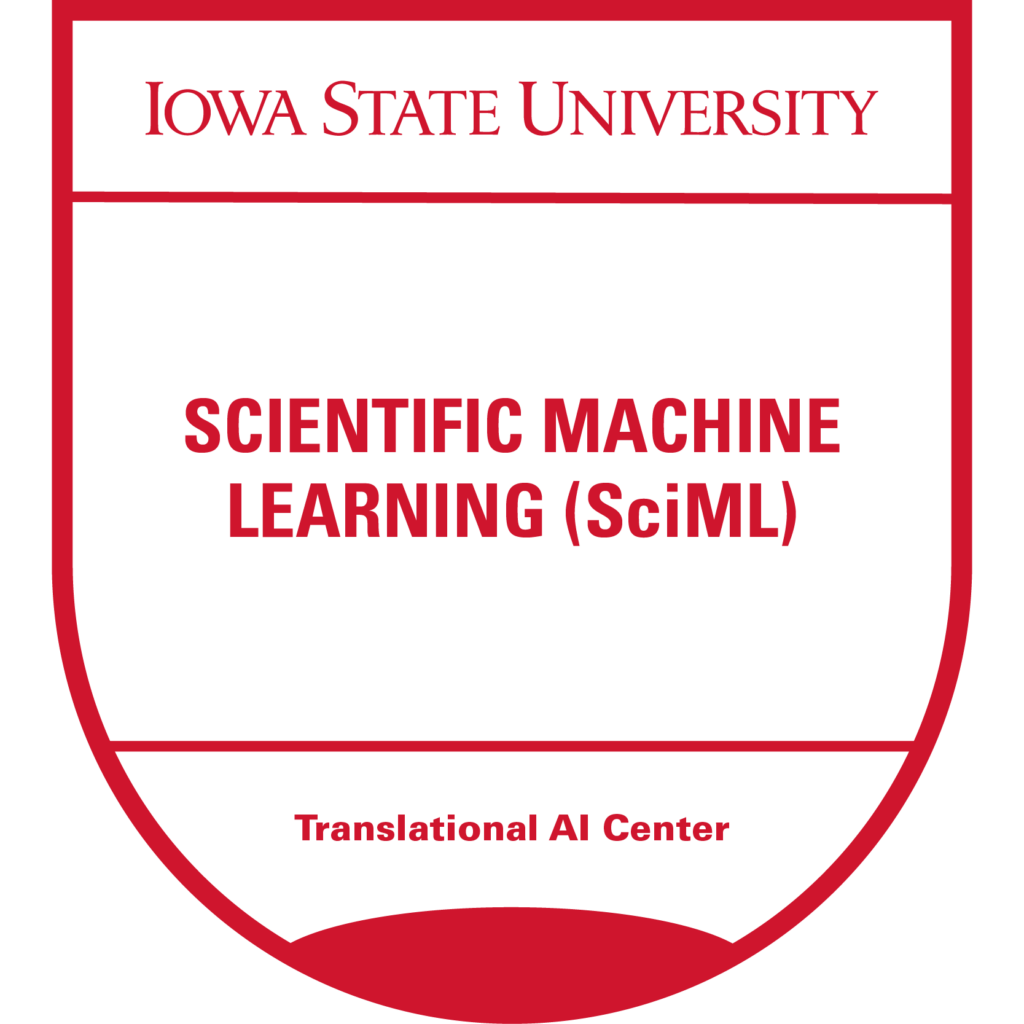
At a Glance
Registration Cost: $750.00 $500 USD (Initial Promo)
*$300 Student & Government Employee
Course Hours: 16 hours
Course Start Date: September 9, 2024
Last Day to Register: September 13, 2024
Instructional Period & Live Sessions: September 3-30, 2024
Last Day to Earn a Micro-Credential Badge: October 31, 2024
Time to Complete Badge: 58 Days
*At the time of registration, you’ll be asked to create an account for this course. Use an email address ending in “.edu” or “.gov” to receive a discount. $200.00 will be immediately credit back after purchase.
Instructor
Ali Rabeh, Postdoctoral Researcher
Ali Rabeh is a postdoctoral researcher at the Translational AI Center (TrAC). He earned his PhD in Mechanical Engineering from Iowa State University. His research interests include scientific machine learning, multiphase flow of immiscible fluids, and finite element methods. He is also exploring the application of state-of-the-art neural operators for modeling fluid flow around complex geometries.